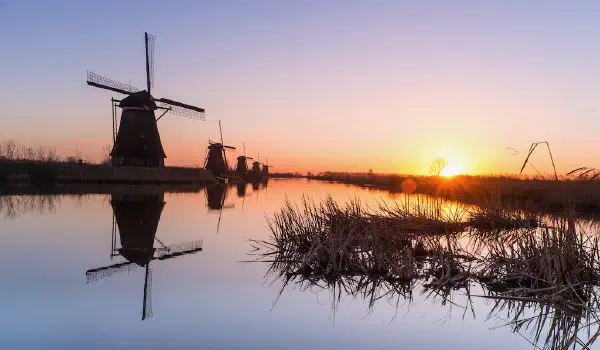
New Version 0.4 of the FI Simulator released
If you have always wanted to know whether factor ETFs or a golden butterfly portfolio allow for earlier financial independence or higher withdrawal rates in retirement, you can now calculate this yourself in the new version 0.4 of the FI Simulator. Furthermore, there are some new analysis features. Here is a list of all new features:
-
In this version a number of new data series have been added. First, there are new US stock indices based on the Factor data by Kenneth French and Eugene Fama. Fama and French use the so-called CRSP database for their research, which is the gold standard for historical stock prices of the US market. Unfortunately, the actual CRSP data is not available to private investors, but fortunately many of the indices of interest to us can be reconstructed with very good precision from the Fama/French data. The procedure required to do this has been described in detail by the author of PortfolioCharts.com here. Kudos to Tyler for this fantastic work! Tyler uses the definition that large cap stocks cover the top 85% of market cap and small cap stocks beyond that cover the 85%-98% portion (the last remaining 2% are micro caps, which are typically left out of a small caps ETF). This is the de-facto standard used by most index providers today. In parallel, the market is divided into half “Growth” and half “Value” stocks depending on the book value / market capitalization ratio. From these 4 sectors, corresponding stock indices can then be constructed, whose performance corresponds very well with the existing factor ETFs in the market today. The big advantage, however, is that these indices constructed in this way start as early as June 1926, i.e. they go back much further than the ETFs that exist today. So in addition to the CRSP All-Caps Index, which tracks the entire U.S. market including micro-caps, we now have new U.S. indices for large cap growth, large cap value, small cap growth and small cap value stocks. The Small Cap Value Index in particular is extremely interesting: since 1926, this index has delivered an excess return over the overall market of over 3 percentage points per year! Another advantage of the new data is that they are available as month-end values and not only as monthly averages as in Robert Shiller’s data. The averages used in Shiller’s data lead to artificially lower volatility and even to measurable deviations of the safe withdrawal rates in critical stock market phases such as during the big depression.
-
In addition to the new CRSP-based US indices, I have also integrated the MSCI World Index as an additional new asset after multiple requests to do so. This index probably represents the portfolio of many current investors much better than pure US or German stock indices could. Thus, the MSCI World would actually be the stock index of choice for the FI-Simulator if only its data would extend a little longer into the past than 1970. If you choose this index for your analyses, you should therefore be aware that you are only using about 50 years of data, which also do not contain many of the critical stock market phases. Therefore, the results should be compared critically with the results obtained from using longer historical data.
-
Last but not least, I have also added gold as another new asset. I am not sure yet if gold can really play a role in a modern retirement portfolio. However, since the data is readily available and goes back a long time, I would like to give everyone the opportunity to analyze this for themselves before settling for a preconceived opinion. When analyzing a “gold-rich” portfolio, however, one must always keep in mind that pre-Bretton Woods 1973 gold prices were pegged to the U.S. dollar, and thus the macroeconomic behavior of this asset pre-1973 is not comparable to post-1973. Nevertheless, it will be exciting to recreate, for example, the Golden Butterfly Portfolio in the simulator. I can already reveal that the results will be surprising.
-
The large number of possible new assets in the portfolio also requires a modification of the asset allocation tab. Now, a checkbox can be set for each asset on the right if this asset is to be used in the optimization and analysis functions below. Unfortunately, the Plotly library I use doesn’t offer a real checkbox within a table, so I currently have to use a small dropdown menu instead. This is not really beautiful, but seems to work okay-ish. As soon as Plotly offers something better, I promise to change this again.
-
Up to now it was possible to calculate a glidepath when optimizing the asset allocation, but the result could not yet be transferred to the own portfolio, because up to now only a fixed asset allocation for an accumulation and a withdrawal phase could be entered there. With the new version it is now finally possible to enter a glidepath length at the bottom. Only if the length of the glidepath is greater than zero, a new column opens up, which allows the entry of a corresponding asset allocation at the beginning of the glidepath. If you are wondering at this point why the column for the asset allocation of the savings phase is missing, please make sure that your FI date is after the simulation start. Only then this column will be shown automatically, otherwise it remains hidden, because then a savings phase does not exist. This hopefully makes the increasingly complex interface a bit more intuitive to use. The other tables below, which can be used to optimize the asset allocation, now behave similarly. Therefore if you are missing columns during input, please always check if glidepath length and FI date are set correctly.
-
The asset allocation optimization functions have been restructured and extended. The new version now allows the separate optimization of the asset allocation during the savings phase (if available), during the withdrawal phase (with a constant asset allocation), during the withdrawal phase with variable asset allocation using a glidepath as well as an optimization of the length of such a glidepath. Last but not least, a fifth sub-tab allows a detailed comparison of the results of a deviating asset allocation with the own “normal” asset allocation.
-
So far the price data used for the calculation of the withdrawal rates was not directly visible anywhere. However, with the above-mentioned addition of many more assets, greater transparency will be helpful in selecting them for one’s portfolio. For this purpose, there is now the new tab “Asset Performance”, which shows the used price charts and calculates the corresponding returns and volatilities for the desired time period. In addition to the “normal” display as logarithmic index charts, there is also the possibility to calculate Telltale Charts made popular by John Bogle. These are especially useful to analyze the factor indices described above in more detail to see in which time periods factors were advantageous.
As with the last update, I have tried again to allow analyses created with the previous version 0.3 to be imported into version 0.4. When saved again, these will be stored in a format compatible with version 0.4. In case of problems with this import I would be grateful for a short hint. In addition, I have again made some changes to the code base under the hood. Therefore, if anyone notices any deviations from the previous version I would again be very grateful for a hint, ideally together with an analysis file (anonymized and freed from critical financial data). Many thanks in advance!
I hope you will like the new features and I look forward to hearing about interesting results you have found with the new data. If you want to be notified about new posts, please use your favorite RSS reader and subscribe to this blog using the RSS link in the main navigation bar or through this link.